Understanding Machine Learning Malware Analysis
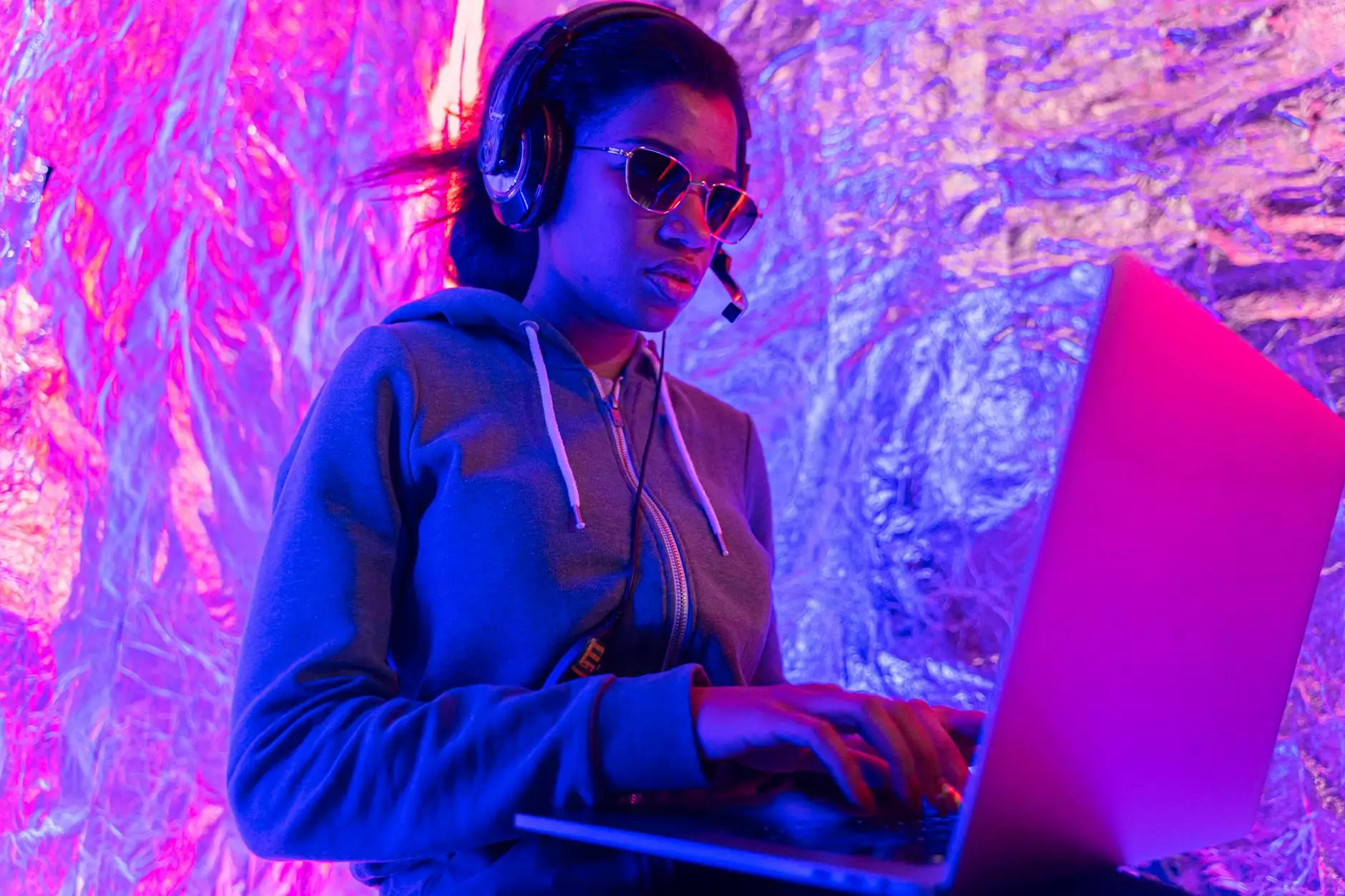
Machine Learning Malware Analysis stands as a crucial pillar in the sphere of cybersecurity, evolving as a significant response to the rapidly changing landscape of threats posed by cybercriminals. As businesses increasingly rely on digital solutions, the threat of malware has correspondingly escalated, necessitating advanced analytical techniques that can anticipate and mitigate these risks.
The Need for Advanced Malware Detection
As technology progresses, malware becomes more sophisticated, making traditional detection methods less effective. This growing risk highlights the urgency for organizations to upgrade their security measures. Here are some reasons why advanced malware detection is essential:
- Increased Volume of Cyber Attacks: On a global scale, cyber attacks occur every 39 seconds, making it imperative for businesses to have robust defense mechanisms.
- Evolution of Malware: Attackers continually evolve their malware, using techniques such as polymorphism and metamorphism to evade detection.
- Data Breaches and Financial Loss: The financial impact of data breaches can be devastating, with the average cost of a data breach reaching millions.
How Machine Learning Enhances Malware Analysis
Machine learning (ML) fundamentally changes how cybersecurity analysts detect and respond to malware. Unlike traditional methods that rely on static signatures, machine learning malware analysis employs algorithms to learn from data and improve detection capabilities over time. Here’s how:
1. Automated Threat Detection
One of the principal advantages of machine learning is its ability to automate threat detection. By training on vast datasets, ML algorithms can identify anomalies that indicate potential threats without human intervention. This automation not only expedites detection but also lowers the costs associated with manual analysis.
2. Continuous Learning and Adaptation
Machine learning systems thrive on data. They continuously analyze incoming data to refine their detection capabilities. This means they can quickly adapt to new malware variants, ensuring that systems remain guarded against new threats. The more data they process, the more accurately they can predict malicious behavior.
3. Behavioral Analysis
ML enables the analysis of software behavior rather than just examining the code itself. By observing how a program behaves within various environments, machine learning algorithms can flag suspicious activities that may not align with typical usage patterns, drawing attention to potential threats that might otherwise go undetected.
Integration of Machine Learning in IT Services & Computer Repair
At spambrella.com, we understand that the integration of machine learning malware analysis in IT services and computer repair is vital not just for detection but also for complete system recovery and protection. Our approach combines cutting-edge technology with expert service to address the challenges posed by modern cyber threats.
Real-Time Monitoring and Response
Businesses require a proactive approach to cybersecurity. By leveraging real-time monitoring through machine learning, we can immediately respond to threats as they arise. This proactive measure minimizes downtime and protects sensitive data from being compromised.
Customized Security Solutions
Every business is different, and so are their cybersecurity needs. Our team conducts thorough assessments to provide tailored security solutions that incorporate machine learning technologies, ensuring each system is fortified against specific vulnerabilities.
Expert Analysis and Consultation
While automation greatly enhances efficiency, the human element remains essential. Our cybersecurity experts analyze machine learning outputs to discern patterns and provide valuable insights that enrich our clients’ overall security posture.
The Role of Security Systems in Protecting Businesses
In tandem with machine learning methodologies, robust security systems form the backbone of any successful cybersecurity strategy. The incorporation of advanced security systems alongside machine learning enhances the overall effectiveness of malware analysis.
Comprehensive Threat Intelligence
A well-informed security system taps into a wealth of threat intelligence. By integrating machine learning capabilities, security systems can utilize real-time data about emerging threats, making them more responsive to new forms of malware.
Multi-Layered Security Approach
A robust security strategy encompasses multiple layers of protection. By pairing machine learning defenses with traditional security protocols like firewalls, intrusion detection systems, and antivirus programs, businesses can create a comprehensive shield against cyber threats.
Challenges and Considerations in Machine Learning Malware Analysis
While the advantages are compelling, machine learning malware analysis does not come without challenges. Organizations must navigate various hurdles to reap its full benefits:
- Data Quality and Availability: High-quality training data is crucial. The scarcity of annotated malware datasets can hinder the efficiency of machine learning models.
- False Positives: Machine learning algorithms may generate false positives, impacting operational efficiency. Balancing sensitivity and specificity is a constant challenge.
- Skill Gap: The demand for skilled professionals who can effectively implement and manage ML systems for malware analysis significantly exceeds the supply.
Future Trends in Machine Learning Malware Analysis
The future of machine learning malware analysis is rife with potential. Emerging trends are set to further revolutionize cybersecurity:
1. Improved Algorithms
As research progresses, new algorithms will enhance detection capabilities. Innovations in neural networks and deep learning provide exciting prospects for future threat detection mechanisms.
2. Enhanced Collaboration
Cross-industry collaboration will lead to the exchange of valuable threat intelligence, allowing machine learning systems to draw from a wider array of data, leading to more effective defenses.
3. Consumer Awareness and Training
As businesses increasingly adopt sophisticated cybersecurity measures, end-user awareness programs will become vital. Training programs to educate employees on recognizing potential threats will complement technological defenses.
Conclusion: Embracing the Future of Cybersecurity
In conclusion, the landscape of cybersecurity is changing. Machine learning malware analysis represents an evolution that is essential for modern IT services and security systems. At spambrella.com, we are committed to staying at the forefront of this technological revolution, providing our clients with state-of-the-art solutions that leverage the power of machine learning to safeguard their businesses.
As the cyber threat theatre grows increasingly complex, businesses must recognize the importance of adopting advanced technologies like machine learning. By doing so, they position themselves to not only defend against current threats but also anticipate and prepare for future ones. Now is the time to invest in your enterprise’s security and embrace the innovations that machine learning has to offer.